Technology Adoption Roadmap for Data Analytics: A 2025 Perspective
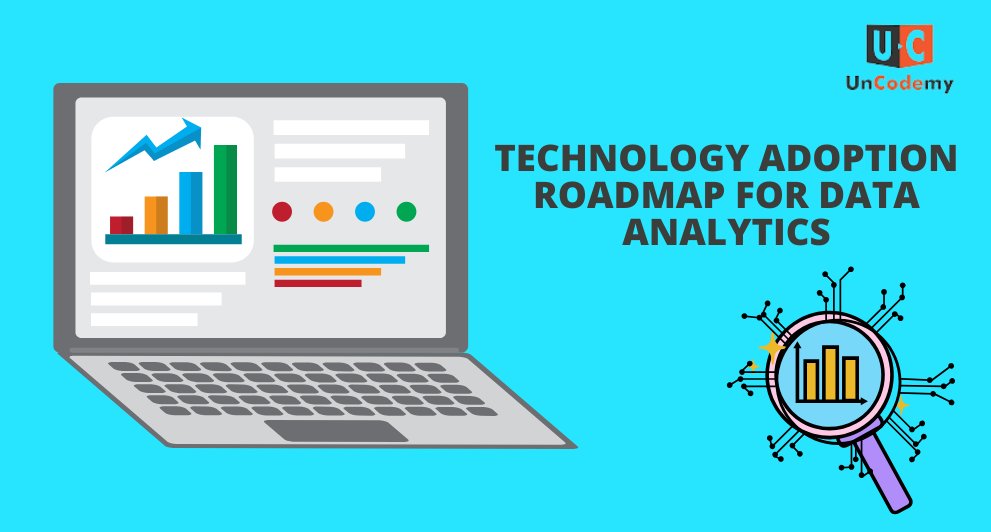
In today’s world, data has become an invaluable asset for businesses across all industries. The evolution of data analytics technologies has been rapid, but so has the complexity of integrating and adopting these technologies. To successfully navigate this dynamic landscape, organizations need a well-defined technology adoption roadmap. This roadmap will help organizations efficiently embrace new tools, systems, and methodologies in data analytics while mitigating risks, ensuring scalability, and maximizing return on investment (ROI).
This article will explore the process of adopting data analytics technologies, focusing on Gartner’s emerging technologies. Understanding these concepts will be pivotal in creating a sustainable adoption strategy for organizations looking to leverage data analytics for their digital transformation journey.
Introduction: The Importance of Data Analytics in Business
Data analytics has transformed from a niche function to a central pillar of business decision-making. Organizations that successfully adopt data-driven strategies are more agile, competitive, and innovative. As data volumes increase exponentially, businesses are faced with the challenge of transforming vast amounts of data into actionable insights.
From predictive analytics to machine learning (ML), artificial intelligence (AI), and advanced data visualization tools, the capabilities of data analytics continue to evolve at an unprecedented pace. Understanding how to adopt these technologies at the right time is crucial to ensuring an organization’s long-term success.
The Gartner Hype Cycle and Technology Adoption Curve
Gartner’s Hype Cycle and Technology Adoption Curve are essential models to help organizations understand the maturity and life cycle of emerging technologies, including data analytics tools. These models provide valuable insights into the evolution of technologies and how organizations should manage their adoption.
The Gartner Hype Cycle
The Gartner Hype Cycle is a graphical representation that depicts the maturity of emerging technologies through five key phases:
- Innovation Trigger: This is the initial phase where a new technology is introduced, generating significant media and industry interest. At this stage, expectations are high, but practical applications and real-world use cases are still in development.
- Peak of Inflated Expectations: In this phase, excitement around the technology peaks. Early adopters push for adoption, but many initiatives fail to meet expectations. Over-hyped capabilities often lead to unmet promises.
- Trough of Disillusionment: After the peak, interest wanes as organizations experience challenges in implementing the technology or fail to see the expected benefits. This phase is characterized by frustration and decreased investment.
- Slope of Enlightenment: In this phase, practical insights are gained, and more realistic use cases are discovered. Early failures lead to a more thoughtful understanding of the technology’s capabilities and limitations. Adoption begins to increase gradually.
- Plateau of Productivity: The technology matures, and organizations start seeing solid benefits. It becomes widely adopted in specific industries or functions, and its potential is fully realized.
Technology Adoption Curve
The Technology Adoption Curve categorizes adopters into different groups based on their willingness to embrace new technologies. These groups include:
- Innovators (2.5%): The first to adopt a new technology, often motivated by a desire for novelty and innovation.
- Early Adopters (13.5%): These are forward-thinking individuals or organizations who see the potential value in new technologies and are willing to experiment, often shaping the future of adoption.
- Early Majority (34%): This group waits until the technology has proven itself to be viable. They tend to be more pragmatic and cautious.
- Late Majority (34%): Late adopters are more skeptical and prefer to wait until the technology is widely accepted and its effectiveness has been demonstrated.
- Laggards (16%): These individuals or organizations are the last to adopt new technologies, often due to a lack of perceived value or resistance to change.
Gartner’s Emerging Technologies 2025: A Glimpse Into the Future
As businesses prepare for the future, it’s important to consider the technologies that will shape the data analytics landscape. Gartner’s 2025 emerging technologies predictions provide a roadmap for what the next generation of tools and capabilities will look like. Below are some of the key technologies highlighted in Gartner’s 2025 list that will significantly influence the data analytics space.
Augmented Analytics
Augmented analytics refers to the use of machine learning, artificial intelligence, and natural language processing to enhance data analytics capabilities. By automating data preparation, insight generation, and decision-making, augmented analytics enables users to uncover insights faster and more accurately than traditional analytics.
Key Benefits:
- Reduced dependency on data scientists and analysts.
- More accurate insights through automated analysis.
- Improved decision-making with actionable recommendations.
Explainable AI (XAI)
As AI and machine learning systems become more integrated into decision-making processes, understanding how these systems arrive at conclusions becomes critical. Explainable AI (XAI) aims to make AI systems more transparent and interpretable, enabling users to understand the rationale behind machine-driven insights.
Key Benefits:
- Increased trust in AI-powered insights.
- Enhanced accountability and compliance with regulations.
- Reduced risk of errors and biases in decision-making.
Cloud Data Platforms
Cloud-based data analytics platforms offer flexibility, scalability, and cost-effectiveness for managing large datasets. The adoption of multi-cloud and hybrid-cloud strategies will continue to rise, allowing organizations to scale their data analytics operations as needed while ensuring high availability and performance.
Key Benefits:
- Scalable and cost-effective infrastructure.
- Seamless integration with other cloud services and tools.
- Enhanced collaboration and accessibility across teams.
Data Fabric
Data fabric is an emerging data architecture that provides a unified view of data across multiple sources and environments. It enables organizations to seamlessly integrate and manage data, irrespective of where it resides.
Key Benefits:
- Simplified data management across complex ecosystems.
- Real-time data access and synchronization.
- Enhanced data security and governance.
Predictive and Prescriptive Analytics
While descriptive analytics (what happened) and diagnostic analytics (why it happened) have been common for years, predictive and prescriptive analytics (what is likely to happen and what should be done about it) are gaining momentum. These technologies utilize advanced algorithms and AI to forecast future trends and recommend actions.
Key Benefits:
- Proactive decision-making based on data-driven predictions.
- Optimization of processes through prescriptive recommendations.
- Improved operational efficiency.
Technology Adoption Lifecycle for Data Analytics
Adopting new data analytics technologies requires careful planning. The technology adoption lifecycle outlines the stages organizations must go through when embracing new technologies, starting with awareness and leading to widespread usage.
Stage 1: Awareness
The first step is to become aware of emerging technologies and understand how they might benefit the organization. During this stage, companies must stay informed through research, attending conferences, and collaborating with technology partners.
- Actions:
- Conduct technology landscape assessments.
- Identify pain points and inefficiencies that data analytics could address.
- Engage with vendors and industry experts.
Stage 2: Evaluation
In this stage, businesses evaluate potential technologies to determine which ones align best with their needs. They also consider factors such as compatibility with existing systems, vendor reputation, and cost-effectiveness.
- Actions:
- Conduct proof-of-concept (PoC) projects.
- Assess potential ROI and scalability.
- Test integration with existing tools and systems.
Stage 3: Pilot
Once the right technology is identified, a pilot program is launched to test its effectiveness in a controlled environment. This allows organizations to evaluate the technology in real-world scenarios before full-scale deployment.
- Actions:
- Define clear objectives for the pilot.
- Involve end-users in the pilot to gather feedback.
- Monitor performance metrics to assess success.
Stage 4: Full Deployment
After successful piloting, the technology is rolled out across the organization. This stage involves scaling the technology, training users, and integrating it with other systems.
- Actions:
- Develop a comprehensive deployment plan.
- Provide training and support for end-users.
- Establish change management strategies to ensure smooth adoption.
Stage 5: Optimization
Once the technology is fully deployed, organizations must continually optimize and improve its usage. This includes monitoring performance, addressing any challenges, and iterating on the adoption process to ensure long-term success.
- Actions:
- Continuously monitor key performance indicators (KPIs).
- Gather feedback from users to enhance functionality.
- Keep up with technology upgrades and updates.
Creating a Technology Adoption Roadmap for Data Analytics
1. Establish Clear Business Goals and Objectives
The first step in creating a technology adoption roadmap is defining the organization’s business goals and understanding how data analytics can help achieve them. Before selecting technologies, ask key questions:
- What specific problems do we need to solve with data analytics?
- What are our long-term business objectives (e.g., improving customer experience, increasing operational efficiency, driving innovation)?
- How will data analytics enhance decision-making and business outcomes?
By clearly defining the objectives, you can ensure that the chosen analytics tools align with the broader strategic direction of the organization.
2. Assess Current Capabilities and Infrastructure
Before implementing new technologies, organizations must take stock of their existing data analytics capabilities. This includes reviewing current data infrastructure, tools, and processes. Key considerations should include:
- Data quality and availability: Is the data clean, structured, and accessible?
- Current technology stack: What tools and platforms are already in use? Do they support new analytics technologies?
- Skills and expertise: Do employees have the necessary skills to leverage new technologies effectively? If not, what training is required?
Conducting a gap analysis of the current state versus desired future capabilities helps in identifying areas that need investment and improvement.
3. Research and Select Relevant Technologies
With a clear understanding of business goals and current capabilities, the next step is researching and selecting the most relevant data analytics technologies. Emerging technologies such as artificial intelligence (AI), machine learning (ML), augmented analytics, and data visualization tools are transforming the landscape. Key technologies to consider include:
- Augmented Analytics: AI and ML-powered tools that automate data analysis, making it easier for non-experts to derive insights from complex data sets.
- Cloud Data Platforms: Scalable and flexible cloud solutions that enable organizations to store, manage, and analyze large volumes of data in real time.
- Predictive Analytics: Leveraging historical data and algorithms to forecast future trends and make proactive decisions.
- Data Fabric: A unified data architecture that simplifies the management of data across various systems and locations.
Look to resources like Gartner’s 2025 Emerging Technologies report to understand the latest trends and technologies shaping the future of data analytics.
4. Pilot Testing and Proof of Concept (PoC)
Once technologies are selected, the next phase is to conduct pilot testing or proof-of-concept (PoC) projects. This allows organizations to test a specific technology on a smaller scale to evaluate its effectiveness and gather feedback before full deployment. In the pilot phase, consider:
- Defining clear objectives: What do you aim to achieve with the pilot? Are you testing the technology’s usability, its impact on business outcomes, or its compatibility with existing systems?
- Engaging key stakeholders: Involve end-users early to ensure the technology meets their needs and to collect valuable feedback.
- Measuring success: Define specific performance metrics (e.g., time saved, insights generated, user satisfaction) to measure the effectiveness of the technology.
A successful PoC allows organizations to make informed decisions about whether to move forward with full deployment.
5. Develop a Phased Implementation Plan
Once the technology is validated in the pilot phase, it’s time to create a detailed, phased implementation plan for full-scale deployment. This plan should prioritize critical use cases and ensure that the deployment is manageable and scalable. A phased approach helps mitigate risks and allows for continuous feedback and iteration. Key considerations include:
- Prioritization of use cases: Focus on high-impact areas that align with business goals. For instance, if the organization’s goal is to improve customer service, prioritize analytics that enhance customer insights.
- Integration with existing systems: Ensure that the new technology integrates seamlessly with existing databases, tools, and workflows.
- Scalability: Plan for scalability as data volumes and user adoption grow. Cloud-based platforms are often preferred for their scalability and flexibility.
A well-defined implementation roadmap helps ensure the project remains on track and is completed within the desired timeframe and budget.
6. Training and Change Management
Adoption of new data analytics technologies often requires a cultural shift within the organization. Change management practices are essential to ensure that the workforce embraces the new tools and processes. Effective change management includes:
- Training programs: Offer hands-on training to ensure employees understand how to use the new tools and interpret the analytics results. Training should be tailored to different user roles, from data scientists to business leaders.
- Building a data-driven culture: Encourage a mindset that values data-driven decision-making at all levels of the organization. Leadership should promote the benefits of adopting data analytics and support employees through the transition.
- Providing support: Implement robust support systems to address user questions and challenges during the adoption process. This might include a helpdesk, user manuals, or peer mentoring programs.
Successfully managing change ensures that employees feel confident in using new analytics tools and understand the value they bring to the organization. For those looking to gain expertise in the Best Data Analytics course in Noida equips individuals with the necessary skills to excel in the field.
7. Monitor and Measure Success
After full deployment, it’s important to continuously monitor the performance and impact of the adopted data analytics technologies. Regular assessments help identify areas of improvement and ensure the technology is delivering on its promises. Key metrics to monitor include:
- User adoption rates: Are employees engaging with the new analytics tools? Are there specific teams or departments that are underutilizing the technology?
- Impact on business outcomes: Track how the technology is influencing key business metrics, such as operational efficiency, revenue, or customer satisfaction.
- System performance: Monitor the technology’s performance, including speed, accuracy, and scalability. Ensure that it’s delivering timely insights without downtime or technical issues.
Ongoing measurement and monitoring allow organizations to fine-tune their approach, ensuring the technology continuously meets evolving business needs.
8. Optimize and Scale
As the organization matures in its use of data analytics technologies, optimization becomes key to ensuring that the systems are being used to their full potential. In the optimization phase, organizations should:
- Enhance functionality: Regularly review the performance of the technology and identify areas where additional features or improvements can be made. For instance, incorporating new data sources or refining algorithms for more accurate predictions.
- Scale as needed: As more data becomes available and user demand increases, ensure that the technology scales to meet these new needs. Cloud-based platforms often offer the flexibility to scale quickly.
- Stay current with updates: Data analytics technologies are constantly evolving. Stay informed about new features, tools, and best practices to ensure that the organization remains competitive and at the forefront of technological advancements.
Optimization ensures that data analytics continues to drive meaningful value over time.
Conclusion
In 2025 and beyond, the adoption of data analytics technologies will continue to shape the way organizations operate, innovate, and grow. By understanding the key elements of the technology adoption lifecycle, leveraging the insights from Gartner’s emerging technologies predictions, and crafting a thoughtful adoption roadmap, businesses can successfully navigate the evolving landscape of data analytics.
The right technology adoption roadmap will ensure that data analytics tools are not just deployed, but embraced and integrated into the fabric of business operations. By doing so, organizations can unlock the full potential of data analytics to drive smarter decision-making, improve operational efficiency, and maintain a competitive edge in the market.
FREQUENTLY ASKED QUESTIONS (FAQs)
1. What is a Technology Adoption Roadmap for Data Analytics?
A technology adoption roadmap for data analytics outlines the strategic steps organizations should follow to successfully integrate new data analytics technologies, from awareness through deployment and optimization. It ensures efficient technology adoption while aligning with business goals and overcoming potential challenges.
2. Why is a Technology Adoption Roadmap important?
A roadmap helps businesses strategically approach technology adoption by providing a clear path, minimizing risks, ensuring alignment with business objectives, and enabling the effective use of data analytics tools. It improves ROI by preventing missteps and ensuring smooth transitions throughout the adoption process.
3. What are Gartner’s emerging technologies for 2025 in data analytics?
Gartner’s 2025 emerging technologies include augmented analytics, explainable AI, cloud data platforms, data fabric, and predictive analytics. These technologies offer advanced capabilities like automated insights, scalable data management, and AI-driven decision-making to enhance business intelligence and operational efficiency.
4. How do the Gartner Hype Cycle and Technology Adoption Curve influence adoption?
The Gartner Hype Cycle tracks the maturity of technologies from initial hype to maturity, helping businesses assess the risks and potential of emerging tools. The Technology Adoption Curve categorizes adopters, from innovators to laggards, providing insight into the adoption stages for planning and resource allocation.
5. What are the phases in the Technology Adoption Lifecycle?
The Technology Adoption Lifecycle includes five phases: awareness (discovering the technology), evaluation (assessing its potential), pilot (testing on a small scale), full deployment (widespread adoption), and optimization (continuous improvement and scaling to maximize value).
6. What role does change management play in technology adoption?
Change management is crucial for ensuring smooth adoption by addressing resistance, providing necessary training, and fostering a culture that embraces technological change. It supports user engagement and ensures that the organization integrates data analytics tools into daily operations effectively.
7. What are the key benefits of augmented analytics in data adoption?
Augmented analytics combines AI and machine learning to automate data analysis and generate actionable insights. It reduces dependency on data scientists, speeds up decision-making, and helps non-technical users interpret complex data, empowering a wider range of employees to contribute to data-driven decisions.
8. How does explainable AI (XAI) impact data analytics?
Explainable AI (XAI) enhances transparency by making AI-driven decisions more understandable. It helps organizations trust machine-driven insights, comply with regulations, and mitigate biases or errors in decision-making, making AI technologies more accessible and accountable.
9. What are the main challenges in adopting new data analytics technologies?
Challenges include data integration across various platforms, selecting the right technology fit, managing change within the organization, ensuring adequate training, and addressing security concerns. A structured adoption roadmap helps mitigate these risks by offering a clear plan for implementation.
10. How can businesses measure the success of their data analytics adoption?
Businesses can measure success by tracking key performance indicators (KPIs) such as user adoption rates, data-driven decision-making frequency, time saved, cost reduction, and improvements in business outcomes like revenue growth or operational efficiency. Regular reviews help optimize performance and ensure alignment with objectives.