What Is Big Data? Definition, Uses, & How It Works Explained
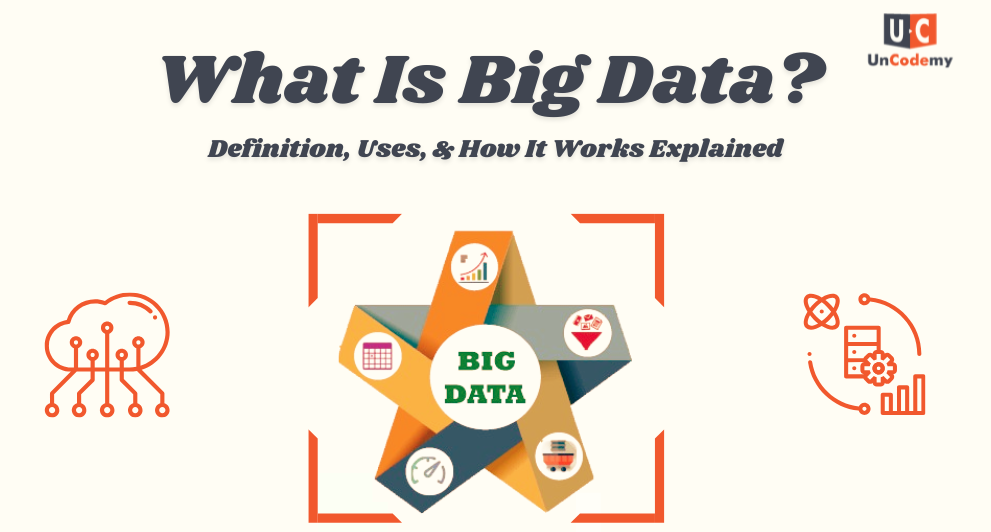
Big Data refers to the massive and ever-growing amount of information generated every day from different sources like social media, sensors, business transactions, and more. This data comes in many formsβorganized data like spreadsheets (structured), messy data like social media posts (unstructured), and everything in between (semi-structured). It’s so large and complex that traditional methods of storing and analyzing data canβt handle it effectively.
Thanks to advancements in technology, including smartphones, the Internet of Things (IoT), and artificial intelligence (AI), the availability of data is increasing rapidly. As the data grows, specialized tools and technologies are being developed to help businesses quickly process and analyze it. These tools allow companies to make better decisions and uncover valuable insights.
In simple terms, Big Data isnβt just about having a lot of data. Itβs about using this data smartlyβwhether itβs for predicting trends, solving problems, or improving customer experiences. For example, Big Data plays a key role in machine learning and advanced analytics, helping businesses make informed decisions and stay ahead in a competitive world.
Examples of Big Data
Big Data can be one of a companyβs most valuable resources. By analyzing it, businesses can uncover important insights about their customers, operations, and market trends. These insights help improve decision-making and drive success.
Here are some simple examples of how Big Data is transforming industries:
- Understanding Customers Better
Companies track how people shop and what they buy to suggest personalized products, making customers feel like recommendations are made just for them. - Stopping Fraud in Its Tracks
By analyzing how customers typically pay, businesses can spot unusual patterns in real-time and prevent fraud before it happens. - Improving Deliveries
Delivery companies combine data about shipping routes, local traffic, and weather to make sure packages arrive faster and more efficiently. - Advancing Healthcare
AI tools analyze messy medical information like doctor notes, lab results, and research reports to discover better treatments and improve patient care. - Fixing Roads
Cities use camera and GPS data to find potholes and prioritize road repairs, making streets safer and smoother. - Protecting the Environment
Satellite images and geospatial data help organizations track the impact of their supply chains on the environment and plan more sustainable operations.
Β
Characteristics of Big Data | The Vs of Big DataΒ Β Β
Big Data is often described using the 3 VsβVolume, Velocity, and Varietyβa concept first introduced by Gartner in 2001. Over time, additional Vs like Veracity, Variability, and Value have been added to capture the full scope of Big Data. Hereβs what each of these means in simple terms:
-
Volume: The Massive Size of Big Data
The sheer size of data is what makes it “big.” Every second, huge amounts of data are generated from devices, sensors, social media, and more. This massive amount of data is what businesses work to collect, store, and analyze.
-
Velocity: The Speed at Which Big Data Moves
Data is being created at an incredible speed. Whether itβs real-time social media updates, sensor readings, or financial transactions, businesses need to process and analyze this data as fast as it comes in to make timely decisions.
-
Variety: The Diverse Forms of Big Data
Data comes in all shapes and forms. It can be:
- Structured: Data neatly organized in tables, like spreadsheets.
- Unstructured: Data like images, videos, or social media posts that donβt fit into a traditional format.
- Semi-structured: Data with some structure, like JSON files or sensor data.
This variety makes analyzing Big Data more complex but also more powerful.
-
Veracity: Ensuring the Accuracy of Big Data
Not all data is reliable. Big Data can be messy, incomplete, or inaccurate, which can make analysis difficult. High veracity means the data is clean, accurate, and trustworthy enough to make good decisions.
-
Variability: How Big Data Context Can Change
The meaning and context of data can change over time. For example, customer preferences may shift, or the type of data collected might evolve based on new trends. This variability makes it challenging to maintain consistency in analysis.
-
Value: Unlocking Insights from Big Data
The most important aspect of Big Data is its ability to provide insights. Businesses need to focus on collecting the right data and analyzing it effectively to uncover patterns, solve problems, and make informed decisions.
How Does Big Data Work?
Big Data is all about using large amounts of information to gain a clearer understanding of situations, spot opportunities, and make better decisions. The idea is simple: the more data you have, the better insights you can uncover to improve your business.
Hereβs how Big Data works, step by step:
-
Integration: Collecting Big Data from Multiple Sources
Big Data comes from many sourcesβwebsites, sensors, social media, and more. All this raw information needs to be collected, processed, and organized into a format that makes sense. This step ensures that analysts and decision-makers can start working with the data.
-
Management: Storing and Organizing Big Data Efficiently
Storing Big Data requires powerful systems because weβre talking about terabytes or even petabytes of data. Companies often use cloud storage, on-premises data centers, or a mix of both. The data must be stored in its raw or processed form and made available quickly, sometimes in real time. Cloud storage is becoming popular because it offers flexibility and can handle massive amounts of data without limits.
-
Analysis: Uncovering Insights from Big Data
The final and most important step is analyzing the data to find valuable insights. This could mean spotting trends, identifying opportunities, or solving problems. Tools like charts, graphs, and dashboards help businesses present these insights in a simple and clear way, so everyone in the organization can understand and act on them.
Big Data Benefits
Big data helps businesses make smarter decisions. By analyzing large amounts of data, companies can find patterns and useful information that guide both day-to-day and long-term decisions.
- Increased Agility and Innovation
With big data, businesses can analyze real-time data and adapt quickly to changes. This helps them launch new products or features faster and stay ahead of the competition. - Better Customer Experiences
By combining different types of data (like customer feedback and behavior), companies can understand their customers better, personalize offerings, and improve overall customer satisfaction. - Continuous Intelligence
Big data allows businesses to gather and analyze data in real-time, constantly discovering new insights and opportunities that help them grow and stay relevant in the market. - More Efficient Operations
Big data tools help companies analyze data quickly, which can reveal areas where they can cut costs, save time, and make their operations run smoother. - Improved Risk Management
By analyzing large amounts of data, businesses can better understand risks and take action to prevent potential problems. This leads to more effective strategies to manage and reduce risks.
Challenges of Big Data Analytics
While big data has many benefits, there are also some challenges that organizations face when working with such large amounts of data. Here are the common challenges:
- Lack of Skilled Professionals
There aren’t enough data scientists, analysts, and engineers who have the skills to manage and analyze big data. These experts are in high demand, making it hard to find the right talent to fully benefit from big data. - Fast Data Growth
Big data grows quickly, and without the right infrastructure in place (for processing, storing, and securing the data), it can become overwhelming to handle. Managing the constant growth of data can be a huge challenge. - Data Quality Issues
Raw data can be messy and disorganized, making it difficult to clean and prepare for analysis. Poor data quality leads to inaccurate insights, which can affect decision-making and business strategies. If not cleaned up properly, the data becomes unreliable. - Compliance and Legal Challenges
Big data often includes sensitive information, which must be handled carefully to meet privacy and legal regulations. Companies need to ensure they follow rules regarding where and how data is stored and processed, such as data privacy laws and regulations. - Integration Difficulties
Data is often spread across multiple systems and departments, which makes it hard to bring everything together. To make the most of big data, organizations must find ways to integrate and connect all the data sources, which can be a complex task. - Security Risks
Big data contains valuable information, making it a target for cyber-attacks. Since the data is diverse and spread across many platforms, protecting it with solid security measures becomes a challenging task.
Β
How Data-Driven Businesses Are Performing
While some businesses hesitate to fully embrace big data due to the time, effort, and resources required to implement it, the benefits of becoming a data-driven organization are clear. Many organizations struggle with changing established processes and adopting a data-first culture, but the payoff is significant.
Hereβs how data-driven businesses are performing:
- 58% of companies that make decisions based on data are more likely to exceed their revenue targets compared to those that donβt.
- Businesses with advanced data insights are 2.8 times more likely to experience double-digit growth year-over-year.
- Data-driven companies typically see over 30% growth annually.
Big Data Strategies and Solutions
Building a solid big data strategy starts with understanding your goals, identifying specific use cases, and evaluating the data you currently have. Youβll also need to figure out if you need additional data and what new tools or systems youβll require to achieve your business objectives.
Unlike traditional data management systems, big data tools are designed to handle large and complex datasets. These tools help manage the volume of data, the speed at which itβs made available for analysis, and the variety of data types involved.
For example, data lakes allow organizations to ingest, process, and store data in its native format, whether itβs structured, unstructured, or semi-structured. They serve as a foundation for running various types of analytics, including real-time analysis, visualizations, and machine learning.
However, it’s important to remember that there’s no one-size-fits-all approach for big data. What works for one company might not suit anotherβs needs.
Here are four key principles to consider when developing a big data strategy:
- Open
Organizations need flexibility to build custom solutions using the tools they choose. As data sources grow and new technologies emerge, big data environments must be open and adaptable, allowing businesses to create the solutions they need. - Intelligent
Big data should leverage smart analytics and AI/ML to save time and improve decision-making. Automating processes or enabling self-service analytics can empower teams to work with data independently, reducing the reliance on other departments. - Flexible
Big data analytics should foster innovation, not limit it. Build a data foundation that offers on-demand access to compute and storage resources. Ensure that your data systems can be easily combined with other technologies to create the best solution for your needs. - Trusted
For big data to be valuable, it must be trusted. This means ensuring your data is accurate, secure, and relevant. Building trust into your data strategy is crucial, and security must be prioritized to ensure compliance, redundancy, and reliability.
Types of Big Data
Big data can be categorized into three main types based on its structure and how it is stored and processed. These types are:
- Structured Data
- Unstructured Data
- Semi-Structured Data
- Structured Data
Structured data refers to data that is highly organized and formatted in a way that makes it easy to store and analyze. It is typically stored in relational databases (RDBMS) or spreadsheets and can be easily processed by traditional data processing tools. Structured data is highly organized, with a predefined model that is easily understandable.
Characteristics of Structured Data:
- Well-organized: Data is arranged in rows and columns.
- Data type consistency: Each column has a specific data type (e.g., integers, strings, dates).
- Relational database format: Stored in tables with defined schemas.
Examples of Structured Data:
- Customer names, phone numbers, and addresses stored in a customer relationship management (CRM) system.
- Financial transactions in banks.
- Product information in an inventory system.
Technologies Used:
- Relational databases (e.g., MySQL, PostgreSQL, Oracle)
- SQL (Structured Query Language)
- Unstructured Data
Unstructured data refers to data that has no predefined structure or organization. It is often difficult to process and analyze because it lacks a consistent format. Most of the data generated today is unstructured, and it often includes rich media like text, images, videos, and more.
Characteristics of Unstructured Data:
- Lacks organization: It does not follow a specific format.
- Complex data types: May include text, images, audio, video, logs, etc.
- Requires advanced processing techniques: Such data cannot be processed using traditional relational databases or tools.
Examples of Unstructured Data:
- Social media posts (tweets, Facebook status updates).
- Emails and messages.
- Audio and video files (e.g., podcasts, videos).
- Log files from servers and applications.
Technologies Used:
- NoSQL databases (e.g., MongoDB, Cassandra, HBase)
- Hadoop and Spark for large-scale processing
- Natural Language Processing (NLP) for text analysis
- Semi-Structured Data
Semi-structured data is a hybrid form of data that does not have the strict structure of structured data, but it still contains some organizational elements that make it easier to analyze compared to unstructured data. This data type often includes tags, markers, or metadata that define elements and their relationships.
Characteristics of Semi-Structured Data:
- Some organization: It may have tags or markers (e.g., XML, JSON, etc.) that provide structure.
- Flexible format: It is not confined to the rigid schema of relational databases.
- Easier to parse and analyze: Semi-structured data can be processed using modern big data tools more efficiently than unstructured data.
Examples of Semi-Structured Data:
- XML files and JSON data (often used in APIs).
- Email with metadata (e.g., sender, recipient, subject).
- Web pages with embedded data (e.g., HTML code).
- Logs from applications in JSON format.
Technologies Used:
- NoSQL databases (e.g., MongoDB, CouchDB)
- XML, JSON parsers
- Hadoop and Spark for processing
Key Components and Techniques in Big Data
Under this heading, the major components and techniques involved in Big Data are:
Β
Big Data Ecosystem and Architecture
The Big Data Ecosystem consists of a variety of interconnected technologies designed to handle the volume, velocity, and variety of data generated across multiple sources. Key components include data storage systems, data processing frameworks, data ingestion tools, and analytics platforms. Data in this ecosystem can range from structured data (like relational databases) to unstructured data (like social media posts, videos, and logs), requiring specialized technologies to manage and process it. The architecture is built around distributed systems, ensuring scalability, fault tolerance, and the ability to handle vast amounts of data efficiently. The architecture typically includes a mix of cloud computing platforms, data lakes, and distributed databases, enabling real-time data access and processing.
- Key Technologies: Hadoop, Apache Spark, NoSQL databases (like MongoDB and Cassandra), data lakes, and cloud platforms.
- Use Cases: From social media analytics to IoT data processing, the big data architecture enables a range of applications in education, healthcare, retail, and more.
- Importance: An effective big data architecture ensures that organizations can scale and innovate without being limited by infrastructure, allowing them to collect and process data from different sources to create meaningful insights.
Hadoop Ecosystem
The Hadoop Ecosystem is one of the most well-known and widely used frameworks for managing and processing big data. Hadoop is designed to handle large-scale data processing and storage through a distributed file system (HDFS). Its MapReduce processing engine breaks large tasks into smaller chunks, processing them in parallel across a cluster of computers. This approach provides high fault tolerance and scalability for big data workloads.
- Core Components:
- HDFS: Distributed storage system that breaks large files into smaller blocks and stores them across a cluster of machines.
- MapReduce: A computational model for processing large datasets in parallel.
- YARN: Resource management system that manages and allocates system resources across the Hadoop cluster.
- Advanced Tools: Tools like Hive, Pig, HBase, and Zookeeper allow users to interact with and process big data using SQL-like languages, data transformation scripts, and real-time data storage solutions.
- Learning Focus: Hadoop is a critical learning tool for anyone interested in big data as it lays the foundation for understanding distributed computing and data processing paradigms.
Apache Spark and Big Data Processing
Apache Spark is an open-source, high-performance big data processing engine known for its speed and ease of use. Unlike Hadoop’s MapReduce, which processes data in batches, Spark allows for in-memory processing, which significantly speeds up the handling of big data. It supports batch, real-time streaming, and machine learning applications.
- Core Concepts:
- RDD (Resilient Distributed Datasets): Spark’s primary abstraction for distributed data, enabling fault tolerance and parallel processing.
- DataFrames and Datasets: High-level abstractions for structured data processing with better optimization and easier syntax compared to RDDs.
- Spark SQL: A component that enables querying data with SQL, making it easier for users to integrate big data with traditional relational databases.
- Use Cases: Spark is used in applications ranging from real-time data analysis (e.g., stock market predictions) to batch processing (e.g., customer data segmentation).
- Learning Focus: Spark is an excellent framework to learn for both data scientists and engineers because of its versatility in handling diverse big data challenges, especially for real-time streaming and machine learning.
NoSQL Databases
NoSQL databases are crucial for storing and managing large amounts of unstructured or semi-structured data, which traditional relational databases (SQL-based) arenβt equipped to handle. They provide flexibility, scalability, and high performance for big data applications.
- Types of NoSQL Databases:
- Key-Value Stores: Simple databases where data is stored as a key-value pair. Examples include Redis and Riak.
- Document Databases: Store data in document format (e.g., JSON or BSON). MongoDB is the most popular document-based database.
- Column-Family Stores: Store data in columns instead of rows, allowing for high performance on analytical queries. Cassandra is a widely used column-family database.
- Graph Databases: Store data as graphs with nodes and edges, ideal for data relationships and networks. Neo4j is a popular graph database.
- Learning Focus: Understanding NoSQL is essential because it helps in working with data that doesn’t fit neatly into tables, like social media posts, IoT data, or logs. It also prepares learners to work with scalable and high-performance database solutions.
Data Ingestion and Integration
Data Ingestion is the process of bringing in data from various sources and formats into a system where it can be processed and analyzed. Since big data environments handle multiple data types, ingestion tools need to be robust and capable of handling high volumes of diverse data in real-time or in batches.
- Tools for Data Ingestion:
- Apache Kafka: A distributed event streaming platform that allows for real-time data ingestion and processing, ideal for IoT data, log data, and other streaming data.
- Apache Flume: A tool for collecting and aggregating log data from various sources, commonly used in web and server log analysis.
- Apache Sqoop: A tool designed to efficiently import data from relational databases into Hadoop ecosystems, useful for ETL (Extract, Transform, Load) processes.
- Learning Focus: Mastering these ingestion tools helps learners understand how to collect, clean, and integrate data from different sources before it can be analyzed or stored in big data systems.
Batch vs Real-Time Data Processing
Batch Processing and Real-Time Data Processing are two primary approaches to processing big data.
- Batch Processing: This approach involves collecting data over a period of time and processing it in chunks. It’s ideal for situations where real-time insights arenβt required. Apache Hadoop and Spark are popular tools for batch processing.
- Use Cases: Historical data analysis, periodic reporting, and data warehousing.
- Real-Time Data Processing: This approach processes data as it arrives, providing immediate insights. This is essential in cases where businesses need to act quickly based on up-to-the-minute data.
- Use Cases: Fraud detection, real-time customer experience optimization, and dynamic pricing in e-commerce.
- Tools: Apache Kafka, Apache Storm, and Apache Flink are popular tools used for real-time streaming and processing.
- Learning Focus: Understanding the difference between these two types of processing is crucial because it dictates how businesses approach their data strategies. Learners should understand which tools and technologies are best suited for their specific processing needs.
Β
Β
Big Data Analytics
Big Data Analytics involves analyzing massive datasets to uncover patterns, correlations, and insights that can inform decision-making. This type of analysis can help organizations make data-driven decisions, improve operational efficiency, and better understand customer behavior.
- Types of Analytics:
- Descriptive Analytics: Helps in understanding past behaviors, patterns, and trends.
- Predictive Analytics: Uses historical data to predict future outcomes.
- Prescriptive Analytics: Provides recommendations on how to handle future actions based on predictive analytics.
- Tools: Apache Hive, Pig, Apache Spark (MLlib for machine learning), Tableau, and Power BI for visualizing insights from big data.
- Learning Focus: Big data analytics is the core of many applications in fields like marketing, finance, healthcare, and education. Learning how to use analytics tools and interpreting results is crucial for anyone working with big data.
Machine Learning with Big Data
Machine learning (ML) with big data refers to the process of using algorithms and statistical models to analyze and draw insights from large datasets. By using big data, ML models can make more accurate predictions, classifications, and recommendations.
- Tools for ML: Libraries like MLlib in Spark, TensorFlow, and Scikit-learn help process big data for machine learning applications.
- Use Cases: Predictive maintenance, recommendation systems, fraud detection, and sentiment analysis.
- Learning Focus: Understanding how to apply machine learning algorithms to big datasets and knowing how to scale and train models on distributed systems is essential for any aspiring data scientist or engineer.
Data Visualization
Data Visualization is the process of representing data in graphical formats to make it easier to interpret and act upon. By visualizing big data, organizations can quickly understand complex datasets and make more informed decisions.
- Tools: Tableau, Power BI, and Qlik allow users to create interactive dashboards and visual reports, enabling decision-makers to explore data trends and insights visually.
- Learning Focus: Mastering data visualization techniques and tools is essential for conveying insights from big data in a way that is easy to understand and actionable for non-technical stakeholders.
Big Data in Cloud Computing
Cloud Computing has revolutionized the way big data is managed by offering scalable, on-demand computing resources. Cloud platforms like Amazon Web Services (AWS), Google Cloud, and Microsoft Azure provide a wide range of services for data storage, computing, and analytics, making them ideal for big data applications.
- Key Services:
- Data Lakes: Store raw, unstructured, and structured data in its native format.
- BigQuery, Redshift, and Azure Synapse Analytics: Cloud-based data warehouses designed to analyze big data.
- Learning Focus: Understanding cloud services and how they support big data environments is essential as more organizations move to the cloud to scale their data processing capabilities.
Security and Governance in Big Data
Security and Governance are critical aspects of big data that ensure data privacy, compliance, and integrity. Organizations need to implement security measures like data encryption, access control, and compliance auditing to protect sensitive information.
- Tools: Security tools like Apache Ranger, Kerberos, and AWS Identity and Access Management (IAM) are essential for securing big data.
- Governance: Data governance involves ensuring that data is accurate, consistent, and properly classified while adhering to compliance regulations like GDPR or HIPAA.
- Learning Focus: Learning data governance and security practices is essential for anyone working with big data to ensure that sensitive data remains secure and compliant with regulations.
Advanced Topics in Big Data
Advanced topics in big data explore the cutting-edge technologies and techniques used to solve complex problems. These include distributed machine learning, deep learning with big datasets, edge computing for real-time processing closer to data sources, and blockchain for data traceability and transparency.
- Emerging Trends: Quantum computing, AI-powered analytics, and edge AI are transforming how big data is processed and analyzed.
- Learning Focus: Students should focus on learning these advanced topics to stay ahead of the curve in the rapidly evolving field of big data and AI.